By Lew Ludwig
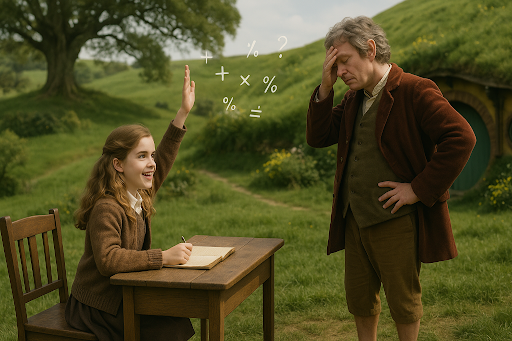
Ten years is a long time to be away from the research table. In that time, I've gained a few gray hairs, lost a few LaTeX shortcuts, and watched the world of generative AI grow from curious whisper to research partner. Lately, I’ve been imagining what it might look like to jump back into undergraduate research after such a hiatus—especially in a world reshaped by tools like ChatGPT and Gemini.
Suppose I had an eager student—let’s call her Hermione Tangent—who approached me with a summer research dream and zero background in knot mosaics. And suppose I, burdened with finals, Center duties, and an inbox full of unread emails, hadn’t kept up with the literature in my once-favorite field. What could we possibly accomplish in eight short weeks?
This imagined scenario gave me a playground to test just how much AI could do—and what it still can’t. Could AI help us jumpstart our journey? Here’s what I found.
The Tools ArXiv Is Already Using
You might not know it, but our trusted friend arXiv is already integrating AI tools into its platform. Scroll to the bottom of a paper and you’ll find tools like:
- Connected Papers, which builds visual networks of related research,
- ScienceCast, which provides AI-generated audio and video summaries, and
- Scite, which adds citation context: who’s building on the work, who’s contradicting it?
These aren’t your standard chatbots. They’re API-powered apps that sit atop large language models, tailored for specific tasks like literature mapping or citation analysis. You don’t have to interact with ChatGPT directly—these tools bring its power to you.
And if you’ve never tried generative AI, these are great on-ramps. No prompt engineering needed, just click and explore.
Putting Gemini to the Test
But I needed something more powerful. Enter Gemini Advanced—Google’s top-tier AI tool. Hermione, still a student, received free access through June 2026 (yes, 2026). So we tried an experiment. I asked her to feed Gemini this prompt:
“I am an undergraduate student in math at a small liberal arts college, finishing my junior year. This summer, I will work with Professor Lew Ludwig to study knot mosaics, a concept introduced by Lomonaco and Kauffman in 2008. Can you create a survey of the work done in knot mosaics to introduce me to the field, plus understand the open questions on this topic?”
About ten minutes later, we had a 22-page survey article. Inside Gemini’s interface, statements like “A central hypothesis put forth by Lomonaco and Kauffman is that knot mosaic theory is equivalent to classical tame knot theory,” came with dropdown citations linking directly to arXiv papers.
Is it 100% accurate? Probably not. I only gave it a reasonable skim. But is it a fantastic starting point for Hermione? Absolutely:
- It gives her a scaffolded overview of the field.
- It links claims to sources.
- It surfaces open questions.
- It orients us both around shared terminology.
- And it saves me dozens of hours I didn’t have.
Putting Manus to Work
Still curious, I tried the same prompt in Manus AI, a fully autonomous agent from the Chinese startup Monica. Manus operates in the cloud (all servers in Singapore), so I wouldn’t put anything sensitive in it. But what it produced amazed me: a 19-page summary similar to Gemini’s—with one hilarious addition.
Section 5.3: Professor Lew Ludwig’s Work
Yes, it stroked my ego by including my contributions. But the real magic came next: it asked if I wanted to turn the paper into a webpage. Five minutes later—boom!—a functional, image-rich webpage summarizing the field.
Were there flaws? Definitely. Some image labels were redundant, and I didn’t check every fact. But as a learning tool for Hermione? Gold. She could review it over break, returning with background knowledge and critical questions. Better yet, it gave her a chance to practice an essential skill: spotting what the AI got wrong.
And that’s no small thing. This is a perfect lesson in automation bias—the all-too-human tendency to overtrust machines, especially when they sound confident. Teaching students to be skeptical, even with well-written AI output, may be just as important as teaching them how to use the tools in the first place.
Summer Is Coming
As you wrap up your spring term and look toward a (hopefully) slower summer, consider taking these tools for a spin:
- SciSpace, which is like a Swiss Army knife for literature reviews and citation work.
- Connected Papers, Scite, or Elicit, all of which can map or critique research for you.
- And if you’re ready to push further, Gemini Advanced and Manus AI have shown real promise—when used with care.
No one asked for this technology, but here it is—uninvited, insistent, and full of possibility. While Hermione is fictional, this thought experiment gave me a glimpse of what it might feel like to return to research with an eager student and a decade's worth of catching up to do. It also showed me how these tools can lower the barrier to entry, making mathematical research more inclusive for curious, motivated students who are just beginning to explore the field. I'm grateful for the space to imagine that scenario and for the tools that help us move a little faster, see a little clearer, and teach a little better. These tools don't just lighten the load—they protect our most limited resources: time, energy, and attention. By handling the repetitive and time-consuming tasks, they free us to focus on the work that matters most: thinking deeply, mentoring well, and maybe even wondering a bit more.
And if I do head back out the research door someday—for real—I’ll be glad to have a guide to help navigate the terrain. After all, even hobbits appreciate a good map when the path is uncertain.
What’s New?
We mathematicians are a thrifty bunch—we love a good bargain and saving a buck. That said, we need to tread carefully with the new AI models. While the idea of shelling out $20 a month for access to these frontier models might seem steep, it's important to recognize that our students have free access to them, thanks to platforms like Google, OpenAI, and Super Grok. Google's Gemini, for example, will remain freely available through June 2026.
If you're trying to make "AI-proof assignments" and only testing them with free models, you might get a false sense of security. The paid models, like GPT-o3 and Anthropic's Claude 3.7 Sonnet, are far more capable, especially in handling mathematical reasoning. An assignment that seems robust and "AI-resistant" when tested with free versions might crumble under the abilities of more advanced models.
So, I encourage you to give these frontier models a try, even if it's just for a month over the summer. Understanding how these advanced tools operate and differ from the free versions will help you better anticipate what your students might use and how they might approach assignments. Plus, you'll gain a clearer picture of the tools' strengths and limitations, allowing you to guide your students more effectively.
Happy exploring—there's a lot to discover!
AI Disclosure: This column was created using the AI Sandwich writing technique.
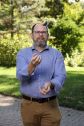
Lew Ludwig is a professor of mathematics and the Director of the Center for Learning and Teaching at Denison University. An active member of the MAA, he recently served on the project team for the MAA Instructional Practices Guide and was the creator and senior editor of the MAA’s former Teaching Tidbits blog.