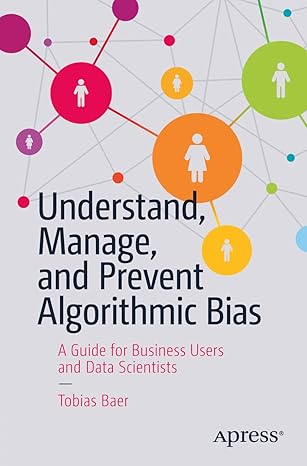
- Author: Tobias Baer
- Publisher: Springer
- Publication Date: 06/07/2019
- Number of Pages: 332
- Format: Paperback
- Price: $54.99
- ISBN: 978-1484248843
- Category: textbook
[Reviewed by Sara Stoudt]
Baer’s Understand, Manage, and Prevent Algorithmic Bias: A Guide for Business Users and Data Scientists provides many actionable and pragmatic approaches to the craft of model building and monitoring at multiple stages of an analysis pipeline.
I went into this book with my own biases. I wondered “can we ever really manage and prevent something as weighty as algorithmic bias?” and feared that this book would contain an over-optimistic collection of false silver bullets. However, even coming from a different context than Baer, I found a lot of common ground in this book.
Baer approaches bias first from a psychological point of view at the individual level and then builds towards more societal level biases. He chooses to discuss societal level biases through a toy alien example that mimics the results of racial disparities but in a potentially less fraught way. At first, honestly, I bristled at this, worrying that it glossed over important issues. However, the advice given in these scenarios was very pragmatic, so I came around to his approach, especially for his target audience explicitly laid out in the title.
Baer’s “artisanal” approach to data analysis avoids undue hype of algorithms; this approach involves separating tasks that can be helped by machine learning and automation from those that humans should still be actively involved in. This “human in the loop” point of view continues, as he stresses the importance of knowing the context of the data when evaluating data inputs and algorithm outputs and recommends talking to people “on the ground” and those involved with the data collection processes.
There is also a good deal of speculative thinking advocated for in the book, including support for constant questioning and looking at the data rather than automatically trusting algorithmic inputs and outputs. What can go wrong? What would it look like if something were to go wrong? Baer illustrates that answering these questions can both inform the investigation of bias and inform checks to avoid those biases in the first place.
Baer’s book also stresses a core value of stability, ensuring there is no breakdown in model performance or increased algorithmic bias when the state of the world changes from the initial conditions the model was trained on. Stability concerns affect not just model building but model monitoring, and Baer connects this value to an explanation of the harms of the feedback loop where algorithms prevent the data needed to correct it, thereby exacerbating the biases. He also explains a helpful concept of “traumatized” data that come from a “disaster” of some sort. The model can learn to connect that region, or those people, with that one “disaster” and the effects can linger in the model, even as the impact on the ground fades (another reason to invest time into understanding the broader context of one’s work).
Although I wouldn’t want this book to close the door on bigger conversations about the root causes of the biases that end up informing and exacerbating algorithmic biases, this book offers guidance that can do a lot of good. Many approaches advocated for in the book require those working with data to pay attention to the broader context and human impact of the decisions they are making throughout their data workflows.
Along with the industry analysts that this book was written for, this book would also be helpful for students thinking about entering industry to give them a sense for what kinds of decisions they may be faced with and helpful steps to go through to help them mitigate the potential harm of those decisions. This book could also serve as a supplementary text for a hands-on statistical or machine learning modeling class at the graduate or upper-level undergraduate level, ideally paired with some readings about the root cause context to give students a fuller picture of how and why these deeper biases can occur.
Data workers must constantly grow in their awareness of the power they and their models wield, but this book provides a strong start for those looking to minimize their blind spots.
Sara Stoudt (https://sastoudt.github.io/) is an assistant professor in the Department of Mathematics at Bucknell University. She is interested in applied statistics and the pedagogy of writing in the STEM fields.