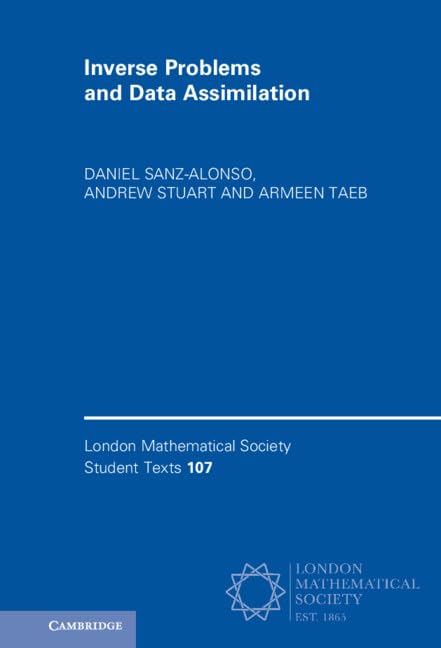
- Author: Daniel Sanz-Alonso, Andrew Stuart, & Armeen Taeb
- Series: London Mathematical Society Student Texts (107)
- Publisher: Cambridge University Press
- Publication Date: 07/01/2023
- Number of Pages: 228
- Format: Paperback
- Price: $39.00
- ISBN: 978-1009414296
- Category: textbook
[Reviewed by Bill Satzer, on 11/13/2024]
Inverse problems and the associated questions of data assimilation are of considerable interest in our data-intensive world. This book offers a concise introduction to these subjects from a mathematical perspective. The authors suggest it is most appropriate for advanced undergraduates and graduate students in mathematics as well as researchers in the sciences and engineering interested in a systematic treatment of methods used in their disciplines.
In brief, inverse problem theory is about how to estimate model parameters from data. More broadly, the subject also addresses the consequences of noisy data, the question of how accurate data must be to enable good estimation of model parameters, and issues of devising strategies for optimization under uncertainty.
By data assimilation the authors are referring to a class of inverse problems where the unknown parameter is the initial condition of a dynamical system or – more broadly – the entire sequence of states for a system with stochastic dynamics. Data assimilation has been most commonly used historically for forecasting – for example, weather forecasting.
The book begins by examining the Bayesian background for inverse problems and considers importance sampling and Markov Monte Carlo methods. The authors describe techniques for approximation methods capable of handling what would otherwise be enormously computationally intensive.
The second part of the book treats the theory of data assimilation. The first two parts of the book have a serial parallel structure so, for example, Bayesian frameworks are described first in both parts. This pattern is then followed in turn for both parts with discussions of the linear setting, optimization, Gaussian approximation, and sampling. A final chapter blends the techniques of inverse and data assimilation to show how Kalman filter data assimilation methods can be applied to inverse problems.
Readers with some background in inverse problems and data assimilation will find good foundational material in this book rigorously presented. In several aspects the subjects are presented elegantly and concisely. However, those with little background are likely to struggle with the intensive theoretical treatment. More concrete examples with real data are sparse.
Some exercises are provided for both inverse problems and data assimilation. The bibliography is very extensive.
A more accessible text is Data Assimilation: Methods, Algorithms, and Applications by Asch et al. This takes a rigorous approach but with a minimum of formalism. It would provide a good point of entry for readers with limited prior background in the area.
Bill Satzer (bsatzer@gmail.com), now retired from 3M Company, spent most of his career as a mathematician working in industry on a variety of applications. He did his PhD work in dynamical systems and celestial mechanics.